The final technical conference program of MATHMOD 2022 is available at https://ifac.papercept.net/conferences/conferences/MAMO21/program/.
Plenary Talks
Mathematical Modeling in Climate Research:
Characteristics and Challenges
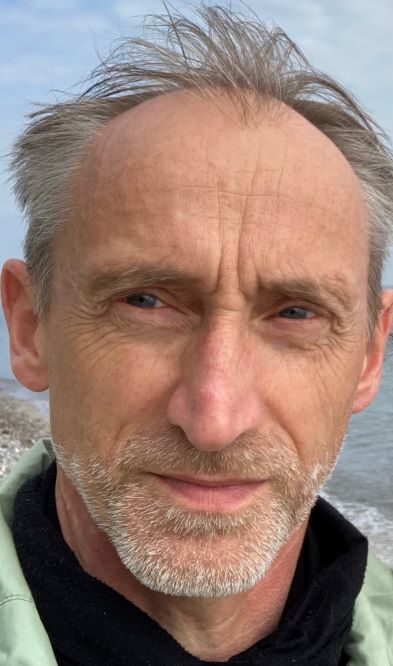
Prof. Thomas Slawig
Leader of the research group Algorithmic Optimal Control — CO2-Uptake of the Ocean, Department of Computer Science, Kiel University, Germany
Climate research and climate predictions heavily rely on models. In this talk, we discuss the underlying modeling principles, specific features and characteristics of climate models, and the resulting consequences for practical applications. The climate system and, thus, also climate models are highly complex. Their evaluation on the computer requires a high computational effort. Moreover, the recent discussions about climate change add some important aspects: The human influence on the climate system results in incorporation of economic and social models, in addition to the classical physical, chemical and biological basis. Vice versa, model results might be used to derive guidelines for political, social and economic strategies. Consequently, reliability of model results and their uncertainty are important issues. Overall, climate modeling and simulation is a highly interdisciplinary topic. This gives rise to look also at the different meanings of the notion “model” in mathematics, computer and climate science.
About the speaker
Thomas Slawig is currently Professor at Kiel University, Germany, where he is leading the research group “Algorithmic Optimal Control – CO2-Uptake of the Ocean”. After earning his PhD in Mathematics in 1998, he has been a scientist at the Potsdam-Institute for Climate Impact Researsch (PIK) and TU Berlin, where he received the “Habilitation” in 2005 . He is a Member of the DFG Excellence Cluster Future Ocean and participates in the German National Climate Modeling Initiative “PalMod: Modeling a complete glacial cycle”.
A Mathematical Diesel Engine Model, its Evolution and Impact on Clean and Efficient Marine Transportation
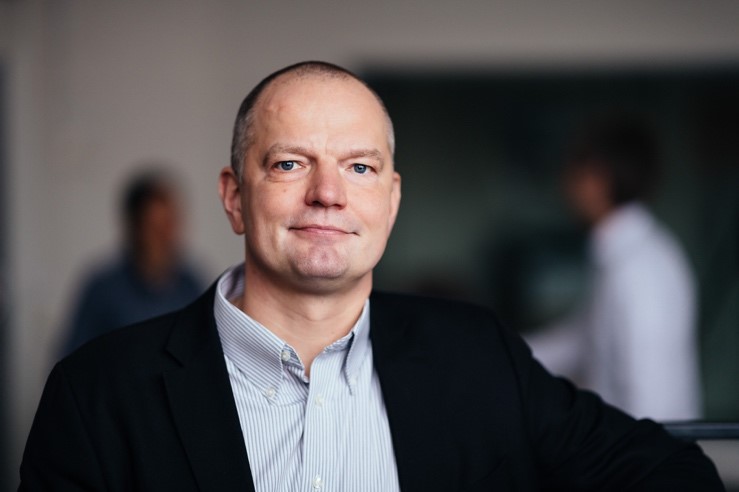
Prof. Lars Eriksson
Professor for Vehikular Systems at Linköping University, Sweden.
Model based development is seen as a key methodology for handling the complexity and guiding the development and optimization of future complex hybrid electric vehicles. It can help reduce the time to market and thus increase the pace of innovation, but a cornerstone for a high innovation pace is the availability and reusability of mathematical models. In this presentation, we will follow the initiation and development of a diesel engine model that has been much used and evolved over the years to become used in a wide range of applications beyond the initial intentions. Starting as a model for a long haulage truck it has been refitted to a passenger car, reused in a diesel electric powertrain in an off-highway application, reused as building blocks for a large marine engine model. Where analysis and adaptive control based on the model has been used to develop clean marine engines fulfilling the UN emission goals. It is now the cornerstone in a benchmark model for development of planning strategies in future connected vehicles as well as in a model for studying hybrid vehicles and how the powertrain interacts with the after-treatment system. Much of the success of the model, builds on the fact that it is component based, systematically developed, and adapted to a real-world engine with documented agreement with measurement data, in addition to that it was released as an open-source model that can be freely downloadable and modified.
About the speaker
Lars Eriksson is Full Professor in Vehicular Systems at Linköping University, Sweden. His research interest is modeling, control, and optimization of clean and efficient vehicle propulsion for sustainable transports. His contributions are foremost on engine control and control-oriented modeling of combustion engines. As the manager of vehicular systems laboratories, he has developed a well-established international network of contacts with research groups both in academia and industry.
Challenges in modelling and detecting the impact of human aptitudes and preferences in economics and finance
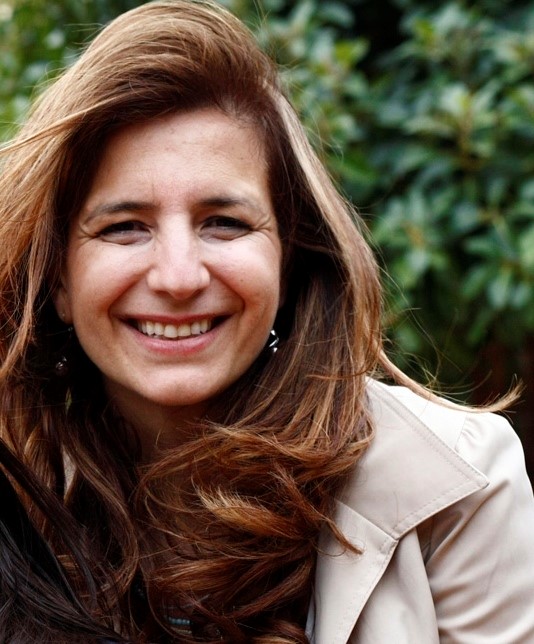
Prof. Marina Dolfin
Professor for Applied Mathematics at the Engineering Department of the University of Messina, Italy.
It is nowadays well-known that the classical deterministic mathematical tools, based on causality principles, generally fail when dealing with the complexity features of behavioral systems. In this talk, I want to address some of these complexity features, i.e. rationality vs. bounded rationality, homogeneity vs. heterogeneity, equilibrium vs. out-of-equilibrium and linearity vs. non-linearity. I will present a toy model to explore the impact of human altruistic vs. selfish aptitudes on the asymptotic wealth distribution of a simulated simple society, as an example to address the aforementioned features using the tools of the kinetic theory of active particles. Finally, I will change the prospective towards the empirical one, presenting an example on detecting lottery-type preferences of investors using market data from the New York Stock Exchange, by means of capital asset pricing models. In this case the emphasis is mostly placed on the role of computation, discussing some aspects related to classical regression vs. machine learning techniques.
About the speaker
Marina Dolfin is Professor at the Engineering Department of the University of Messina (Italy) in Applied Mathematics. She is a visiting lecturer since the 2017 and currently PhD at the King’s Business School of the King’s College London (UK) in complex systems in economics and finance.
Evening Plenary Lecture
Landscape Archaeology as an Interface between Natural Sciences and Humanities
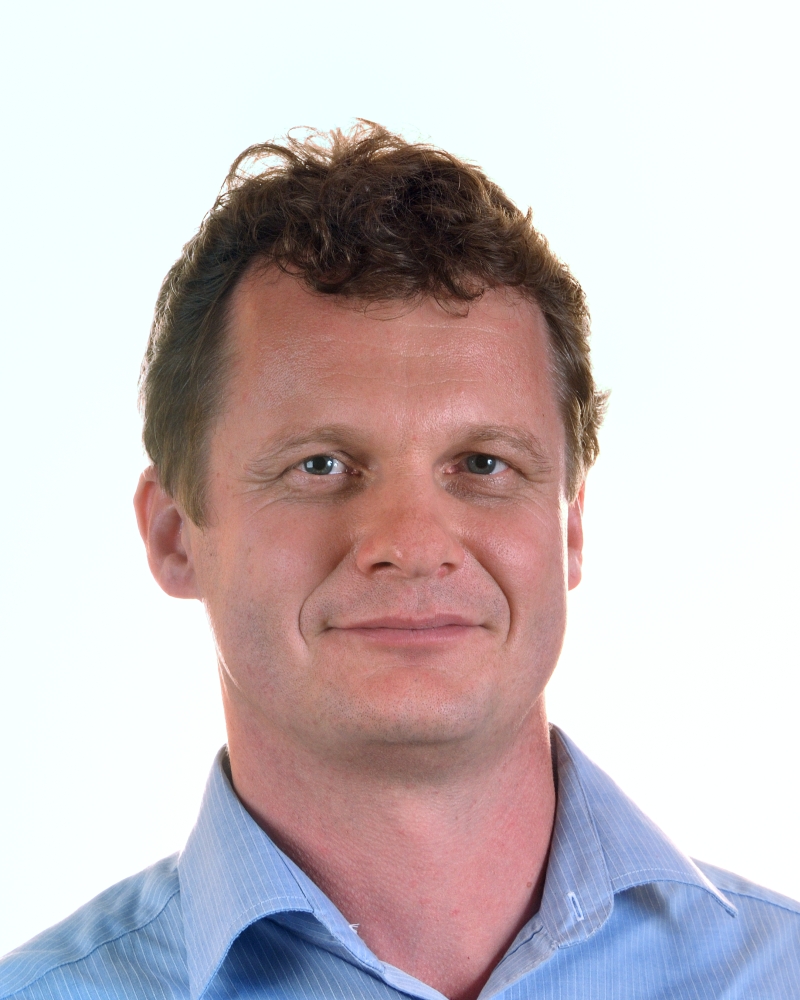
Prof. Michael Doneus
Professor for landscape and environmental archaeology at the Department of Prehistoric and Historical Archaeology, University of Vienna, Austria
Archaeological methodology has undergone major changes in recent decades. For the past century, archaeology has been mainly associated with digging, and excavation was seen as the primary archaeological information source. Today, this view is being challenged by the rapid development and application of a broad spectrum of non-invasive approaches, including airborne remote sensing and geophysical prospection techniques such as magnetometry and ground penetrating radar. In particular, the widespread use of an integrated array of large scaled archaeological prospection techniques has shifted the focus from an artefact-based interest in single sites to a completely new view that allows for a more comprehensive understanding of cultural landscapes. The evening lecture will give an insight into the techniques and methodologies of archaeological prospection and demonstrate its contribution to the discovery and interpretation of past societies and their landscapes.
About the speaker
Michael Doneus is professor for landscape archaeology and Head of the Department of Prehistoric and Historical Archaeology of the University of Vienna. He is specialized in archaeological prospection with a focus on methodological development of remote sensing techniques and integrated data interpretation. During the past decade, he was also deputy director of the Ludwig Boltzmann Institute for Archaeological Prospection and Virtual Archaeology and director of the Vienna Institute for Archaeological Science.